Is Artificial Intelligence Widening the Diversity Gap in Hiring Practices? [Part 1]
Published: Jun 28, 2019
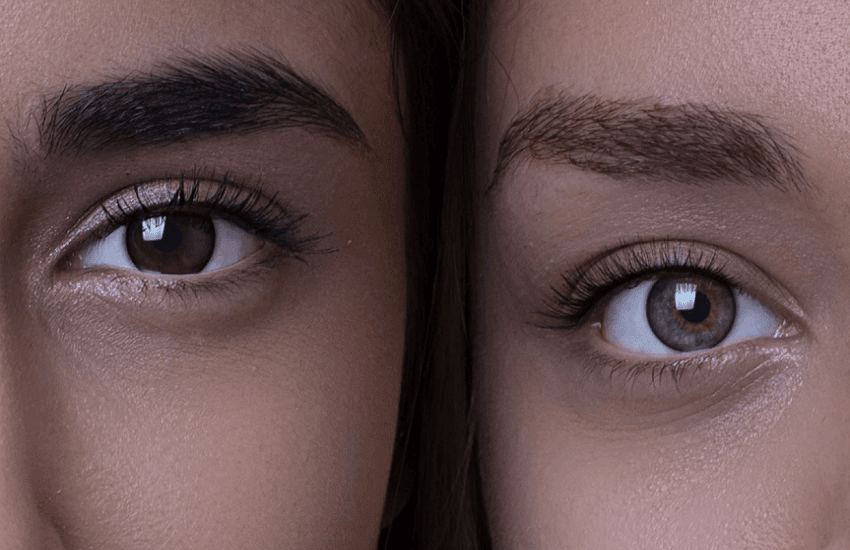
Advances in artificial intelligence (AI) are driving the future of businesses. This technology promises a seemingly endless number of possibilities and myriad implications for virtually all aspects of running a company. One of those implications is the recruitment of new talent, as HR teams across different industries are leveraging the power of AI to assist in their recruiting efforts. But are these developments helping or hurting businesses? Moreover, how do candidates fare against an AI-driven recruiting system?
Vault recently spoke with Vladimir Sidorenko, founder and CEO of international personnel management company Performia CIS, about how AI is used in recruitment. Below, he discusses how AI can actually contribute to the diversity gap in hiring efforts—particularly in the tech industry—and how HR professionals can foster diversity and better utilize AI to maximize profit for their companies.
Vault: How has AI revolutionized the way businesses recruit new talent? What do you think is the biggest implication AI has for HR professionals?
Sidorenko: Artificial intelligence in recruiting allows for computer systems to develop problem-solving skills to streamline recruiting efforts. An example would be software that applies machine learning to review resumes and filter out candidates. Sentiment analysis of job descriptions can identify personally biased language. When used effectively, AI can save recruiters time by automating tasks for HR.
Right now, 79 percent of recruiters’ time is spent on searching for the right candidate. It can at times feel impossible for teams to find the right candidate for their business. However, there have been advances in artificial intelligence that have assisted in the hiring process—such as natural language analysis—which can go beyond looking at a candidate’s resume and consider a person’s online presence. This can be an indicator of how a person can fit into the company culture, and it can change a whole scope of business as a company can accurately target the right candidate.
Vault: What are the biggest challenges facing companies that leverage AI in their recruiting efforts?
Sidorenko: A majority of AI applications are based on the category of algorithms known as deep learning and how it finds patterns in data. In the tech industry, we are seeing how human bias can seep into these systems, especially within recruiting. For example, Amazon abandoned a recruiting algorithm after it showed that it favored men’s resumes over women’s. Microsoft’s AI services had an error rate of 21 percent when asked to analyze darker faces, while 35 percent error rates occurred for IBM’s facial recognition technology. Right now, it is clear that gender and racial biases occur in AI.
Vault: Would you say this is—at least in part—what accounts for the significant gap in the representation of women and minorities in the tech industry?
Sidorenko: Yes, I think gender and racial bias in AI plays a substantial role in the gap between men, women, and minorities in tech. 22 percent of AI professionals are female while 78 percent are male. Also, reports show that 85 percent of AI projects will deliver erroneous outcomes due to bias in data, algorithms or teams managing them. But, I think it also comes down to the lack of fair representation and inclusion of women and minorities within the workforce. HR teams need to put additional emphasis on philanthropic investments and programs that empower and connect people from all walks of life.”
Vault: How, exactly, does AI “learn” to be biased? Is this a result of human error—human biases—on the part of those who have developed these technologies?
Sidorenko: This is a very complex issue. I think this article in MIT’s technology review sums it up well. To paraphrase, computer scientists can create a deep learning model to decide what business or HR objective they want to achieve. However, there are consequences to this, as these models are built to solely reach one objective, not accounting for fairness or discrimination. These algorithms can show gender and racial bias in the attempt to reach the objective that it was designed to reach.
Biases can show up in data that doesn’t represent reality or shows an existing prejudice. Deep learning algorithms can show more photos of light-skinned faces than dark-skinned faces, which would be a fault in the facial recognition system, but existing prejudices—like Amazon’s recruiting tool—were dismissing female candidates because their module was trained to evaluate historical hiring choices, which were not favorable to women.
AI bias is hard to fix because deep learning processes do not consider bias detection and the ways computer scientists are taught to frame problems lack a social context. These problems can leave fairness excluded from the process. Overall, the deep learning algorithms still need programming to identify and eliminate bias, but a diverse workforce can provide a useful hand in outlining social context that can be inputted into deep-learning algorithms.
Click here for part two of our conversation.
--
Vladimir Sidorenko is the Founder and CEO of Performia CIS, an international personnel management consulting company. Created in 2001, they specialize in effective solutions to personnel problems and technology for hiring productive employees and contributing to higher profits for companies. Performia International is headquartered in Stockholm, Sweden and Performia CIS (Commonwealth of Independent States) is located in Moscow, Russia.